Join Us at the 2nd AI Spring School
A three-day event, hosted by UTSA MATRIX, NSF NAIAD, ATHENA, and the UTSA Office of Research! Dive into the latest advancements in AI and machine learning (ML) for edge systems. Discover how cutting-edge neuro-inspired and distributed (federated) algorithms are enhancing energy efficiency and robustness in AI. Learn from top experts from Google, NSF, CMU, Duke, and more. Engage in hands-on activities and connect with the vibrant AI/ML community. Open to all students and researchers, this event is your opportunity to deepen your knowledge and shape the future of AI at the edge. Don’t miss out on this exciting journey!
Invited Distinguished Speakers
Araceli Ortiz, PhD ▼
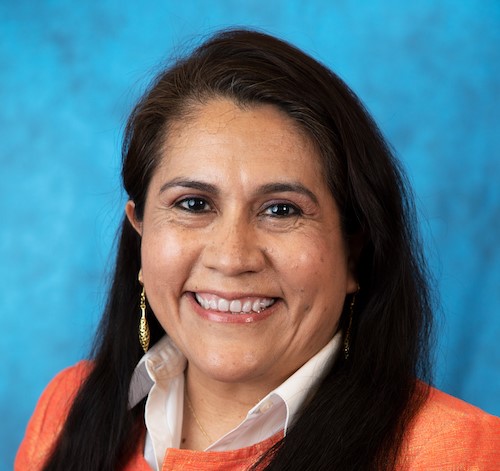
Date: February 18, 2025
Time: 4:15 – 5:15 PM
Institution: University of Texas at San Antonio
Presentation Title: Cultural Competence and Artificial Intelligent Systems: Opportunities, Challenges, and Considerations
Abstract:
As Artificial Intelligence (AI) systems increasingly influence decision-making across diverse sectors, it is critical to ensure cultural competency in their design and application. This tutorial explores fundamental theories of social psychology, culture, and technology and considers the significance of cultural competency for AI designers, researchers, and educators. For technical professionals, achieving cultural competency requires a structured approach to dataset curation, algorithmic transparency, and human-centered design. This tutorial briefly overviews practical methodologies to ensure AI systems serve all populations well. Additionally, educators will gain insights into integrating cultural competency into AI curricula.
Biography:
Dr. Araceli Martinez Ortiz is the Microsoft President’s Endowed Professor of Engineering Education at the Klesse College of Engineering and Integrated Design, University of Texas at San Antonio. She is the Principal Investigator of multiple externally funded engineering education research efforts. She serves as the Executive Director of the Manuel P. Berriozábal Pre-Freshman Engineering Program (PREP) and Program Director of Engineering Education Graduate Education. Dr. Martinez Ortiz earned a bachelor’s degree in Industrial and Operations Engineering from the University of Michigan in Ann Arbor. She also holds Masters degrees in Management from Kettering University and Education from Michigan State University and received her Ph.D. in Engineering Education from Tufts University. With a robust background of fifteen years in engineering and technical industries and another fifteen years in research and education, Araceli now leads national intervention and research efforts that explore the impact of strengths-based, integrated content and culturally responsive instructional and design approaches. She collaborates with colleagues specializing in education, engineering, and artificial intelligence and leads projects aligned with strategic priorities and funding from NASA, the NSF, and the NIH. Several of her engineering education intervention programs have been implemented nationwide, helping thousands of students recognize their potential and develop technical skills for future engineering careers.
Igor Bilogrevic, PhD ▼
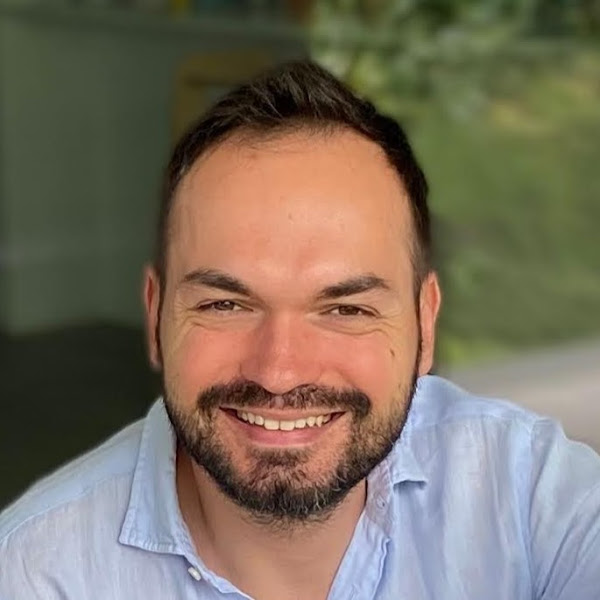
Date: February 18, 2025
Time: 9:30 – 10:30 AM
Institution: Google
Presentation Title: Enhancing Safety on the Web with On-Device ML
Abstract:
The web is a powerful platform for developers and users, enabling the former to provide personalized experiences and sophisticated services to the latter. Most users experience the open web through their browser, which is their agent in the online world. Safety is a critical aspect in the online world, and browsers have different ways to mitigate online threats. In this talk, I will cover two recent advances in web safety: notifications spam mitigation and distributed browser fingerprinting detection. Most notification prompts are not granted by users, but they constitute a potential threat vector for subsequent online abuses (e.g., phishing). To decrease the notification spam in Chrome, we designed, evaluated and deployed an on-device ML model that decides whether to show a less visible permission prompt depending on the browsing context and the users’ past actions in such context. Regarding browser fingerprinting, which is a privacy-invasive technique to extract a quasi-unique device identifier from the browser’s properties and device characteristics, we designed and evaluated a novel detection approach based on federated learning with differential privacy guarantees.
Biography:
Dr. Igor Bilogrevic is a Staff Research Scientist working to bring novel machine learning and AI features for privacy and security in products. His mission is to make technology simpler, safer and smarter for the users. By default. He holds a PhD on applied cryptography and machine learning for privacy-enhancing technologies from EPFL (Switzerland). Previously, he worked in collaboration with the Nokia Research Center on privacy challenges in pervasive mobile networks, encompassing data, location and information-sharing privacy. In addition, he spent a summer at PARC (a Xerox Company), conducting research on topics related to private data analytics. Dr. Bilogrevic is a co-inventor on several patents filed by Nokia, PARC and Google. He is interested in several domains that are related to the applications of machine learning and AI to privacy and security, such as web browser privacy and contextual intelligence.
John Basl, PhD ▼
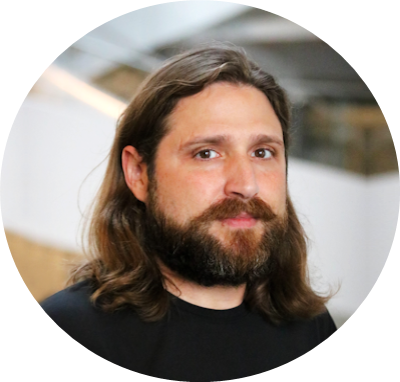
Date: February 19, 2025
Time: 1:00 – 3:00 PM
Institution: Northeastern University
Presentation Title: Value-Analysis in Design: Tools and Techniques for Integrating Ethics in Emerging Technology Research
Abstract:
This tutorial will introduce participants to Value Analysis in Design (VAD). VAD is a framework and toolkit for identifying and resolving ethical issues in technology research, design, development, and deployment. The tutorial will provide an overview of the framework, some of the lenses by which we can evaluate emerging technologies, and then have participants engage in a number of activities to develop tools for value analysis.
Biography:
Dr. John Basl is an Associate Professor of Philosophy at Northeastern University and an Associate Director of the Northeastern Ethics Institute where he leads AI & Data Ethics Initiatives. He works in AI ethics, the ethics of emerging technologies, and moral philosophy. He is co-ethics lead of the National Internet Observatory and Co-PI of the NSF-funded Summer Training Program to Expand the AI and Data Ethics Research Community.
Joshua (Jovo) Vogelstein, PhD ▼
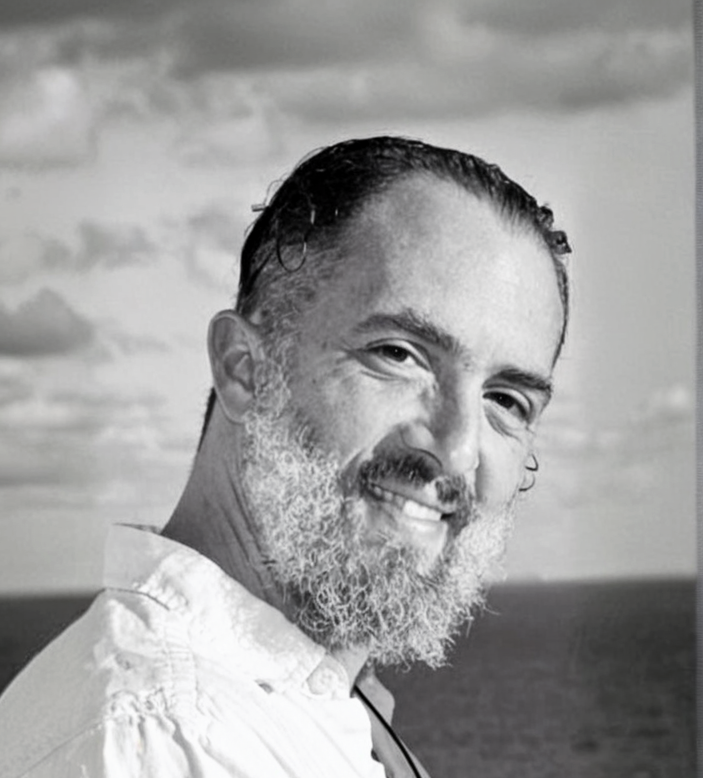
Date: February 18, 2025
Time: 9:30 – 10:30 AM
Institution: Johns Hopkins University
Presentation Title: It’s About Time: Learning in a Dynamic World
Abstract:
In real-world applications, the distribution of the data, and our goals, evolve over time. The prevailing theoretical framework for studying machine learning, namely probably approximately correct (PAC) learning, largely ignores time. As a consequence, existing strategies to address the dynamic nature of data and goals exhibit poor real-world performance. This paper develops a theoretical framework called “Prospective Learning” that is tailored for situations when the optimal hypothesis changes over time. In PAC learning, empirical risk minimization (ERM) is known to be consistent. We develop a learner called Prospective ERM, which returns a sequence of predictors that make predictions on future data. We prove that the risk of prospective ERM converges to the Bayes risk under certain assumptions on the stochastic process generating the data. Prospective ERM, roughly speaking, incorporates time as an input in addition to the data. We show that standard ERM as done in PAC learning, without incorporating time, can result in failure to learn when distributions are dynamic. Numerical experiments illustrate that prospective ERM can learn synthetic and visual recognition problems constructed from MNIST and CIFAR-10. Finally, we extend these results to learning with control, including a foraging problem.
Biography:
Dr. Jovo is an Associate Professor in the Department of Biomedical Engineering at Johns Hopkins University, with joint appointments in Applied Mathematics and Statistics, Computer Science, Electrical and Computer Engineering, Neuroscience, and Biostatistics. His team’s research focuses primarily on the intersection of natural and artificial intelligence. They develop and apply high-dimensional nonlinear machine learning methods to biomedical big data science challenges. Having published about 200 papers in prominent scientific and engineering venues, with ~15,000 citations and an h-index 54, his group is one of the few in the world that regularly publishes in both top scientific (e.g., Nature, Science, Cell, PNAS, eLife) and top artificial intelligence (e.g., JMLR, Neurips, ICML) venues. They have received funding from the Transformative Research Award from NIH, the NSF CAREER award, Microsoft Research, and many other government, for-profit and nonprofit organizations. Jovo has advised over 60 trainees, and taught about 200 students in his eight years as faculty. In addition to his academic work, he co-founded Global Domain Partners, a quantitative hedge fund that was acquired by Mosaic Investment Partners in 2012, and software startup Gigantum, which was acquired by nVidia in early 2022. He lives in the Chesapeake Bay Watershed with his beloved eternal wife and their three children.
J.R. Rao, PhD ▼
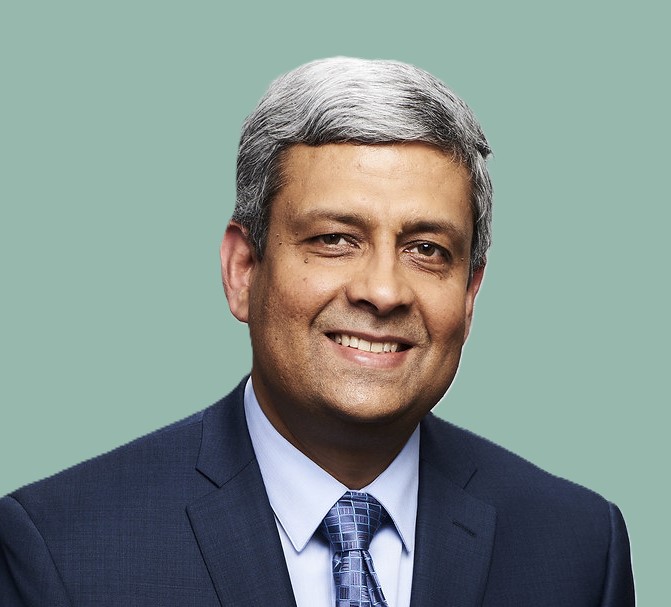
Date: February 17, 2025
Time: 2:00 – 3:00 PM
Institution: IBM Research
Presentation Title: Securing the Enterprise AI Frontier: A Framework for Protecting Foundation Models in Practice
Abstract:
As AI transitions from consumer applications to enterprise deployments, organizations face unprecedented challenges in securing foundation models and generative AI systems. This talk explores the critical security, privacy, and compliance requirements that must be addressed to enable widespread enterprise adoption of AI technologies. With foundation models demonstrating increasingly sophisticated capabilities in content generation, the need for robust security measures has never been more urgent. We will examine the emerging threat landscape surrounding generative AI, including risks related to AI infrastructure, data, model, and applications. We will outline comprehensive strategies for securing AI models throughout their lifecycle – from development and training to deployment and monitoring. Drawing from experiences at IBM and industry-wide initiatives, we will discuss practical approaches to implementing security controls, ensuring data privacy, maintaining regulatory compliance, and establishing governance frameworks for enterprise AI systems.
Biography:
Dr. J.R. Rao is an IBM Fellow and CTO, Security Research for IBM. The Security Research team comprises over 200 researchers working in areas such as Cybersecurity, AI Security, Cloud and Systems Security, Information Security and Cryptography. JR works closely with commercial and government customers, academic partners, and IBM business units to drive new and innovative technologies into definitive industry standards and IBM’s products and services. The goal of his research is to significantly raise the bar on the quality of security while simultaneously easing the overhead of developing and deploying secure solutions. He has published widely and holds numerous US and European patents. He obtained his Doctorate degree from University of Texas at Austin, a Master’s degree from State University of New York at Stony Brook, and a Bachelor of Technology degree from Indian Institute of Technology, Kanpur.
Rahul Bhargava, MS ▼

Date: February 19, 2025
Time: 10:45 – 11:45 AM
Institution: Northeastern University
Presentation Title: AI for Good: Applications in the Pro-Social Sector
Abstract:
Local governments, newsrooms, civil society organizations, and others are racing to partner with technologists to thoughtfully apply emerging AI to their real-world problems. This requires aligning goals across sectors that are sometimes at odds. In this talk, I’ll introduce methods and concrete examples that help offer a more inclusive path to AI adoption in the pro-social sector. Key principles include building with, not for, communities, studying up to interrogate structures of power, using the minimal effective tool for any job, addressing hidden risks of long-term AI integration, and focusing on underserved problems as new opportunities. These serve as inspirations for finding pathways to meaningful, lasting innovation with new AI technologies in the civic space.
Biography:
Rahul Bhargava is an educator, researcher, designer, and facilitator who builds collaborative projects to interrogate our datafied society with a focus on rethinking participation and power in data processes. He has created big data research tools to investigate media attention, built hands-on interactive museum exhibits that delight learners of all ages, and run over 100 workshops to build data culture in newsrooms, non-profits, and libraries. Rahul has collaborated with a wide range of groups, from the state of Minas Gerais in Brazil to the St. Paul library system and the World Food Program. His academic work on data literacy, technology, and civic media has been published in journals such as the International Journal of Communication, the Journal of Community Informatics, and been presented at conferences such as IEEE Vis and ICWSM. His museum installations have appeared at the Boston Museum of Science, Eyebeam in New York City, and the Tech Interactive in San Jose.
Xaq Pitkow, PhD ▼
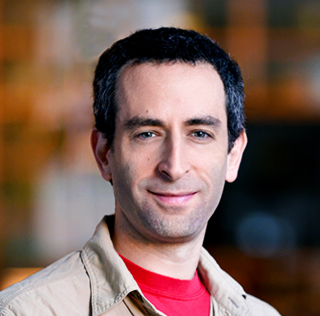
Date: February 17, 2025
Time: 1:00 – 2:00 PM
Institution: Carnegie Mellon University
Presentation Title: Principles of Constrained Intelligence
Abstract:
A key measure of intelligence is the ability to generalize beyond data. Machine learning has made enormous strides recently, yet our best algorithms still fail to generalize the way humans do, and even their successes are built on voracious hunger for data and energy. I’ll outline key principles that contribute to better generalization that rationally trade-off costs of accurate inference and control. We’ll apply this to the famous system of LQG control, with Linear dynamics, Quadratic costs, and Gaussian noise, and reveal a new family of suboptimal but rational behaviors, where you move more to think less. This work provides a foundation for a new type of rational computations that could be used by both brains and machines for efficient but computationally constrained control.
Biography:
Dr. Xaq Pitkow studies general mathematical principles of intelligent systems, both natural and artificial. He was trained in physics as an undergrad at Princeton, went on to study biophysics for his PhD at Harvard, and did postdocs at Columbia and University of Rochester in theoretical neuroscience. He spent a decade in Houston as a faculty member at the Baylor College of Medicine and Rice University, and then moved to the Carnegie Mellon University, appointed in the Neuroscience Institute with a courtesy appointment in the Department of Machine Learning. He is currently the Associate Director of the NSF AI Institute for Artificial and Natural Intelligence.
NAIAD Speakers
Aaditya Khant (Doctoral Student) ▼
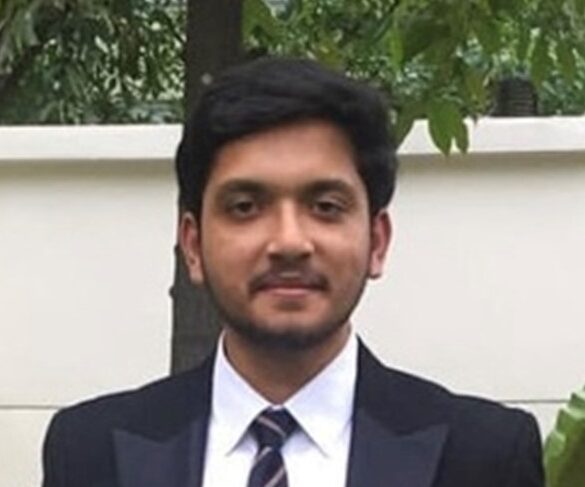
Date: February 18, 2025
Time: 10:45 – 11:45 AM
Institution: University of Texas at San Antonio
Presentation Title: Spiking Neural Networks-based Audio Fidelity Evaluation
Abstract:
Recent advancements in generative AI have enabled the creation of highly realistic synthetic audio, posing significant challenges in voice authentication, media verification, and fraud detection. While Artificial Neural Networks (ANNs) are frequently used for fake audio detection, they often struggle to generalize to unseen and complex manipulations, particularly partial fake audio, where real and synthetic segments are seamlessly combined. The presentation shows the use of Spiking Neural Networks (SNNs) for fake and partial fake audio detection – an unexplored area. Study included comprehensive evaluations encompassing hyperparameter tuning, cross-dataset generalization, noise robustness, and frame-level partial fake audio detection using multiple large-scale public audio datasets. SNNs achieved performance comparable to state-of-the-art ANN models while showing better generalization capabilities and robustness to noise.
Biography:
Aaditya Khant is a doctoral student in the Computer Science department at UTSA. After earning his master’s degree from UT Dallas, he joined UTSA in 2024 and has been a member of the SPRiTE lab ever since. His research focuses on advancing artificial neural networks, with a particular emphasis on neuro-inspired spiking neural networks and neuromorphic data.
Anurag Daram (Doctoral Student) ▼
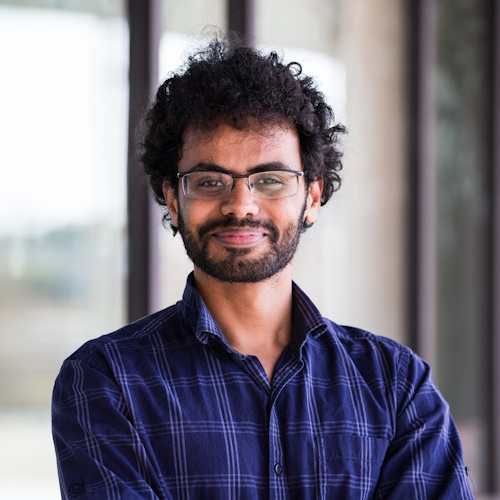
Date: February 18, 2025
Time: 2:00 – 3:00 PM
Institution: University of Texas at San Antonio
Biography:
Anurag Daram received his B.Tech. degree in Information and Communication Technology from DA-IICT, India, in 2016 and Masters in Computer Engineering from Rochester Institute of technology, USA, in 2019. He joined the Neuromorphic Artificial Intelligence Lab in 2017 and is currently a PhD candidate in Electrical Engineering at University of Texas at San Antonio. His research interests include developing energy-efficient AI algorithms, and hardware-software co-design architectures for learning on the edge.
Dhireesha Kudithipudi, PhD (Program Organizer & Host) ▼
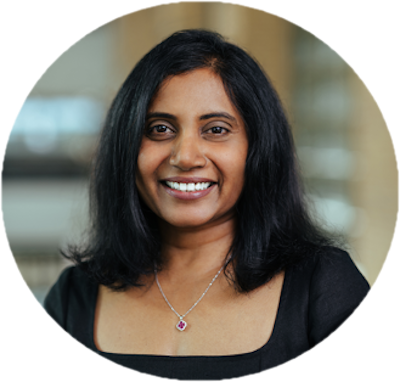
Date: February 18, 2025
Time: 1:00 – 2:00 PM
Institution: University of Texas at San Antonio
Presentation Title: THOR: The Neuromorphic Commons — A National Hub
Abstract:
The Neuromorphic Commons (THOR) project aims to accelerate research innovation by creating a large-scale neuromorphic computing resource. By partnering with leading neuromorphic companies and providing open-source software frameworks and benchmarks, THOR will drive advancements in algorithm design, hardware/software co-design, and neuromorphic applications. Researchers from the University of Texas at San Antonio, the University of Tennessee Knoxville, the University of California San Diego, and Harvard University are involved in developing and deploying this infrastructure. THOR will offer community access to diverse neuromorphic hardware systems, enhancing understanding of computational models, algorithms, and neuromorphic hardware, and supporting research in neuroscience and bioinspired processing applications.
Biography:
Dr. Kudithipudi, founder of the MATRIX AI Consortium, is a trailblazer in neuro-inspired AI, designing systems that mimic biological intelligence. She compassionately leads large multidisciplinary AI teams, including hundreds of scientists, with transparency and adaptability, fostering a culture of innovation. She initiated the country’s first MD/MS program in AI and heads national centers of excellence focused on human well-being and energy-efficient AI. As a first-generation student and the first PhD graduate of Klesse College, Dr. Kudithipudi is paving the way for others to succeed and thrive.
Hai (Helen) Li, PhD ▼
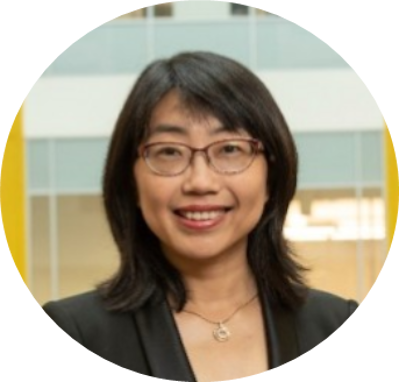
Date: February 17, 2025
Time: 9:30 – 10:30 AM
Institution: Duke University
Presentation Title: Enhancing Efficiency, Privacy, and Safety of Large Language Models at Edge
Abstract:
In this talk, we explore cutting-edge strategies for optimizing large language models (LLMs) on edge devices, focusing on three pivotal aspects: efficiency, privacy, and safety. We first delve into the efficiency of LLM inference and training, highlighting state-of-the-art (SOTA) techniques in quantization, mixture of experts (MoE), and innovative backpropagation-free training approaches designed for edge constraints. On the privacy front, we address the critical issue of personally identifiable information (PII) leakage in LLMs under malicious attacks, presenting a novel unlearning method to mitigate privacy risks. Lastly, we discuss the safety of pre-trained models, particularly in training data detection, and showcase our advancements in identifying instances where generated content stems from pre-training data. We hope this session offers a comprehensive look at the challenges and solutions for deploying secure, efficient, and private LLMs at the edge.
Biography:
Dr. Hai (Helen) Li is the Marie Foote Reel E’46 Distinguished Professor and Department Chair of the Electrical and Computer Engineering Department at Duke University. She received her B.S. and MS degrees from Tsinghua University, and her PhD degree from Purdue University. Her research interests include neuromorphic circuits and systems for brain-inspired computing, machine learning acceleration and trustworthy AI, conventional and emerging memory design and architecture, and software and hardware co-design. Dr. Li served/serves as the Associate Editor-in-Chief and Associate Editor for multiple IEEE and ACM journals. She was the General Chair or Technical Program Chair of multiple IEEE/ACM conferences and the Technical Program Committee member of over 30 international conference series. Dr. Li has received many awards, including the IEEE Edward J. McCluskey Technical Achievement Award, Ten Year Retrospective Influential Paper Award from ICCAD, TUM-IAS Hans Fischer Fellowship from Germany, ELATE Fellowship, nine best paper awards, and another ten best paper nominations from IEEE/ACM. Dr. Li is a fellow of IEEE, ACM, and NAI.
Miroslav Pajic, PhD ▼
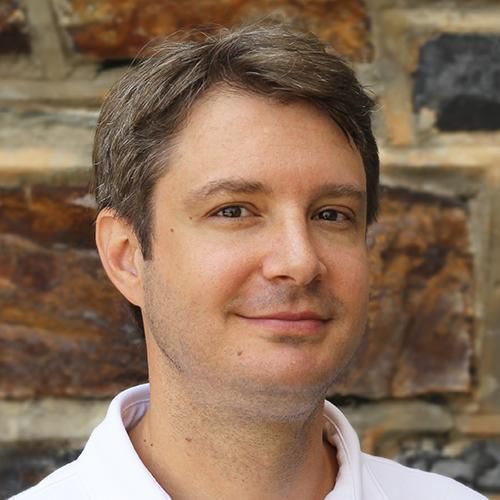
Date: February 19, 2025
Time: 3:45 – 4:15 PM
Institution: Duke University
Presentation Title: dge Autonomy in Contested World
Biography:
Miroslav Pajic is Professor in the Department of Electrical and Computer Engineering at Duke University. He also holds a secondary appointment in the Computer Science Department, and the Department of Mechanical Engineering and Material Science. His research interests focus on the design and analysis of high-assurance cyber-physical systems with varying levels of autonomy and human interaction, at the intersection of (more traditional) areas of AI, learning and controls, embedded systems, robotics and formal methods. Dr. Pajic received various awards including the NSF CAREER Award, ONR Young Investigator Program Award, ACM SIGBED Early-Career Researcher Award, IEEE TCCPS Early-Career Award, IBM Faculty Award, ACM SIGBED Frank Anger Memorial Award, the Joseph and Rosaline Wolf Dissertation Award from Penn Engineering, as well as eight Best Paper and Runner-up Awards, such as the Best Paper Awards at the 2017 ACM SIGBED International Conference on Embedded Software (EMSOFT) and 2014 ACM/IEEE International Conference on Cyber-Physical Systems (ICCPS), and the Best Student Paper award at the 2012 IEEE Real-Time and Embedded Technology and Applications Symposium (RTAS). He is an associate editor in the ACM Transactions on Cyber-Physical Systems and ACM Transactions Computing for Healthcare (ACM HEALTH), and was a Chair of the 2019 ACM/IEEE International Conference on Cyber-Physical Systems (ICCPS’19).
Murtuza Jadliwala, PhD (Program Organizer & Host) ▼
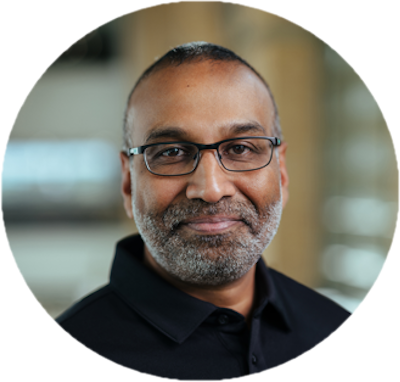
Institution: University of Texas at San Antonio
Biography:
Dr. Murtuza Jadliwala is an Associate Professor and Cloud Technology Endowed Fellow in the Department of Computer Science at the University of Texas at San Antonio, USA. Prior to that, he was an Assistant Professor in the Department of Electrical Engineering and Computer Science at the Wichita State University, USA from 2012-2017 and a Post-doctoral Research Fellow in the Department of Computer and Communication Sciences at the Swiss Federal Institute of Technology in Lausanne (EPFL) from 2008-2011. He also served as a Summer Faculty Fellow at the US Air Force Research Lab – Information Directorate in Rome, NY, USA from June-August 2015. His educational background includes a Bachelors degree in Computer Engineering from Mumbai University, India and a Doctorate degree in Computer Science from the State University of New York at Buffalo, USA. His research in cyber security and privacy has been funded with grants and awards from the National Science Foundation (NSF), US Air Force Office of Scientific Research (AFOSR), Air Force Research Lab (AFRL) – Information Directorate, National Aeronautics and Space Administration (NASA) and Power Systems Engineering Research Center (PSERC). He received NSF’s CAREER Award in 2020.
Panagiotis Markopoulos, PhD (Program Organizer & Host) ▼
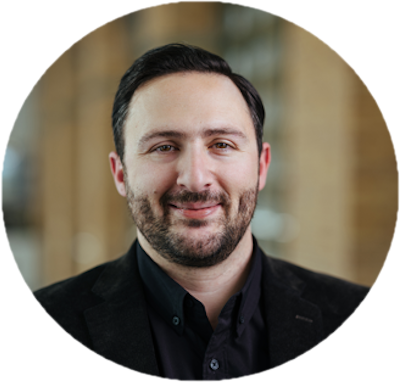
Date: February 19, 2025
Time: 3:15 – 3:45 PM
Institution: University of Texas at San Antonio
Presentation Title: Federated Learning in Healthcare: A Brief Review of Foundations, Applications, Challenges, and Opportunities
Zoom Link: https://utsa.zoom.us/j/99388370235
Abstract:
Federated Learning (FL) is transforming artificial intelligence by enabling decentralized model training across institutions without requiring direct data sharing. This talk will begin with a brief review of FL’s foundational principles, structural components, and key methodologies, emphasizing its role as a privacy-preserving machine learning paradigm. We will then explore some key applications of FL in healthcare, including real-time patient monitoring, personalized treatment, broader medical research, and AI-driven drug discovery. Current implementations and studies demonstrate FL’s ability to enable multi-institutional collaboration while maintaining data privacy, allowing for improved model generalization, greater resource efficiency, and enhanced decision-making in clinical practice and research. We will also review some of the challenges FL must overcome to maximize its benefits to health systems, such as data heterogeneity, model convergence issues, communication overhead, generalization limitations, bias, and computational costs, which further complicate clinical adoption. As research advances and these challenges are addressed, FL has the potential to revolutionize collaborative AI in medicine, driving secure, data-driven healthcare innovation while safeguarding patient privacy.
Biography:
Dr. Panagiotis (Panos) P. Markopoulos is an Associate Professor and Margie and Bill Klesse Endowed Professor in the Departments of Electrical & Computer Engineering and Computer Science at The University of Texas at San Antonio (UTSA). He is the Founding Director of the UTSA Machine Learning Optimization (MILO) Laboratory and Co-Lead for Trustworthy AI at MATRIX: The UTSA AI Consortium for Human Well-Being. Dr. Markopoulos is an expert in machine learning, with a research focus on efficient and robust learning from challenging data (e.g., corrupted, limited, distributed), multimodal deep learning, federated machine learning, and quantum machine learning. His work has major applications in remote sensing, wireless communications, and healthcare, among other fields. He has authored over 80 research publications and has been awarded significant external funding from agencies such as the National Science Foundation (NSF) and the Air Force Office of Scientific Research (AFOSR), including the YIP award. Among other projects, he is also currently conducting research for the NIH/AIM-AHEAD project MATCH: The MATRIX AI/ML Concierge for Healthcare, which aims to develop an open AI/ML platform for clinicians in Texas and internationally, providing tools and training for the interpretation of diverse biomedical and healthcare data.
Rakib Ul Haque (Doctoral Student) ▼
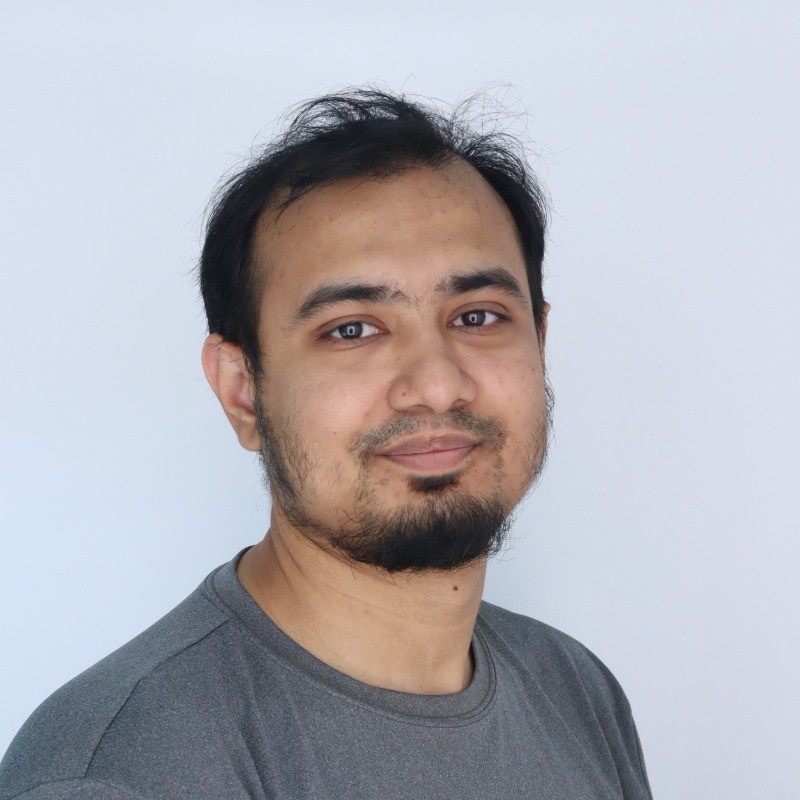
Date: February 18, 2025
Time: 10:45 – 11:45 AM
Institution: University of Texas at San Antonio
Presentation Title: Local and Global Hyperparameter Tuning for Efficient Federated Learning.
Abstract:
Federated Learning (FL) is a distributed machine learning paradigm where multiple clients collaboratively train a global model across multiple update rounds without sharing their local data. FL has numerous applications in healthcare, defense, and other domains. Selecting hyperparameters such as learning rate and batch size is a crucial yet challenging task. Poor hyperparameter selection can lead to slow accuracy improvements and an excessive number of update rounds, which is particularly problematic since each round incurs substantial communication, computation, and energy costs. Existing adaptive tuning methods are often domain-dependent and sensitive to initialization. In this work, we introduce a new approach that dynamically tunes hyperparameters both locally (at the clients) and globally (at the server), promoting faster convergence while reducing communication and energy overhead. Extensive experiments on computer vision tasks demonstrate the effectiveness of this method.
Biography:
Rakib Ul Haque is currently pursuing his doctoral studies and working as a graduate research assistant at The University of Texas at San Antonio (UTSA). He holds an MS degree in Computer Science and Technology from the University of Chinese Academy of Sciences, Beijing, China. Prior to his doctoral studies, he served as a lecturer at Shanto-Mariam University of Creative Technology in Dhaka, Bangladesh. Additionally, he has contributed as a reviewer for several journals and conferences. His research interests encompass deep learning, federated learning, and neuro-inspired machine learning.
Spencer Hallyburton (Doctoral Student) ▼
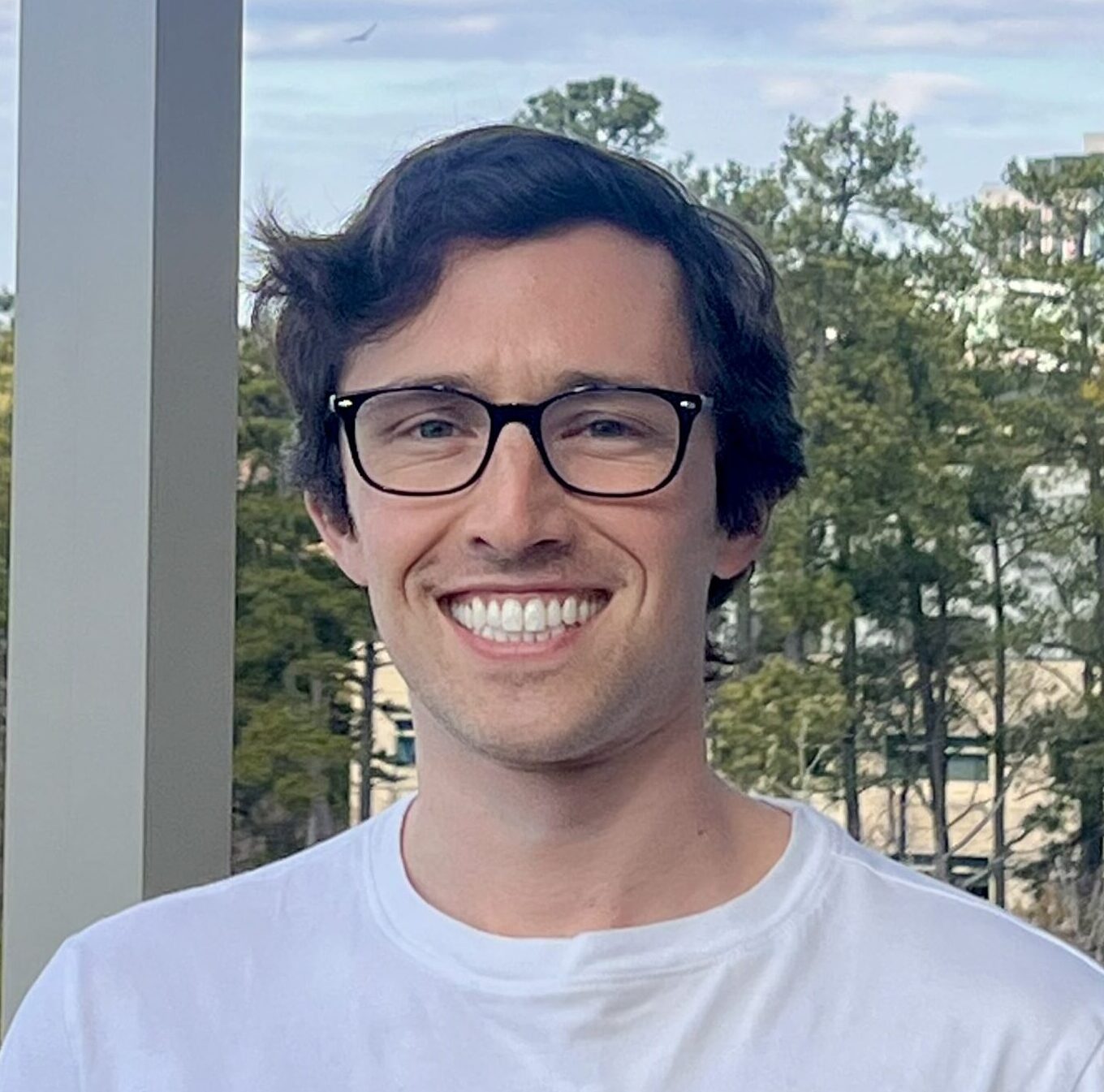
Date: February 18, 2025
Time: 10:45 – 11:45 AM
Institution: Duke University
Presentation Title: Trust-Based Security-Aware Sensor Fusion in Autonomy
Abstract:
Lacking security awareness, sensor fusion in multi-agent networks such as smart cities is vulnerable to attacks. This work introduces a trust-based framework for assured sensor fusion in multi-agent networks, utilizing a hidden Markov model (HMM) to estimate the trustworthiness of agents and their provided information. Trust-informed data fusion prioritizes fusing data from reliable sources, enhancing resilience and accuracy in contested environments. To evaluate assured sensor fusion under attacks on sensing, we present a novel multi-agent dataset built from the Unreal Engine simulator, CARLA.
Biography:
Spencer Hallyburton is a doctoral candidate in Duke University’s Cyber-Physical Systems lab investigating assured autonomy. Spencer’s security analysis of perception algorithms led to his discovery of the frustum attack, a major vulnerability of multi-sensor fusion. In response, he is diving deep into novel methods for trust-based and neuro-symbolic security-aware sensor fusion. He is the primary author of AVstack, an open-source platform for module-level and full-stack autonomous vehicle design and analysis. To guide his research, he employs diverse mathematical and applied techniques including estimation theory, deep learning, and Bayesian statistics.
Birds Of A Feather
Amanda Fernandez, PhD ▼
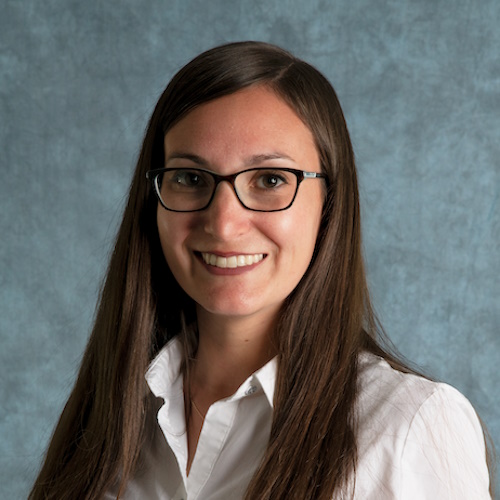
Institution: University of Texas at San Antonio
Biography:
Dr. Amanda Fernandez is an Assistant Professor in the Department of Computer Science at the University of Texas at San Antonio. Prior to joining UTSA, she worked in industry as a software engineer and machine learning researcher. She holds a Bachelor’s degree in Computer Science from Siena College in NY, and both a Master’s and Doctorate degree from the University at Albany SUNY. Dr. Fernandez is recognized as a Senior Member of the IEEE and the National Academy of Inventors (NAI). At UTSA, she serves as a MATRIX AI Consortium Thrust Lead for Machine Learning & Deployment, and as the Faculty Advisor to the ACM-W and GDSC student organizations. Her research in deep learning and computer vision has been funded by grants and awards from the National Science Foundation (NSF), U.S. Department of Energy (DOE), and U.S. Department of Defense (DOD).
Fred Martin, PhD ▼
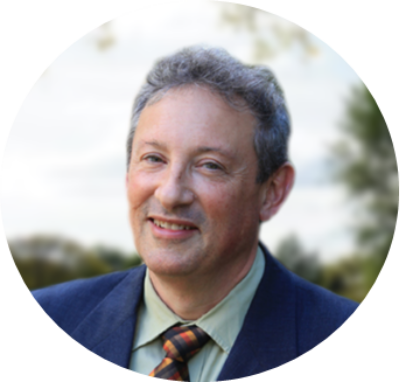
Institution: University of Texas at San Antonio
Biography:
Dr. Fred Martin invents and studies new technologies to enable teaching and learning in computer science, data science, and artificial intelligence. He creates partnerships for bringing these technologies to learners in school and out of school. Focusing on K-12 teachers and students, he collaborates with researchers in other fields, particularly in education and psychology. Martin received the 2022 AAAI/EAAI Outstanding Educator Award for his contributions to the Artificial Intelligence for K-12 Initiative (aik12.org). He is also a past chair of the Computer Science Teachers Association (CSTA) and currently a professor and chair of computer science at The University of Texas at San Antonio.
Kevin Desai, PhD ▼
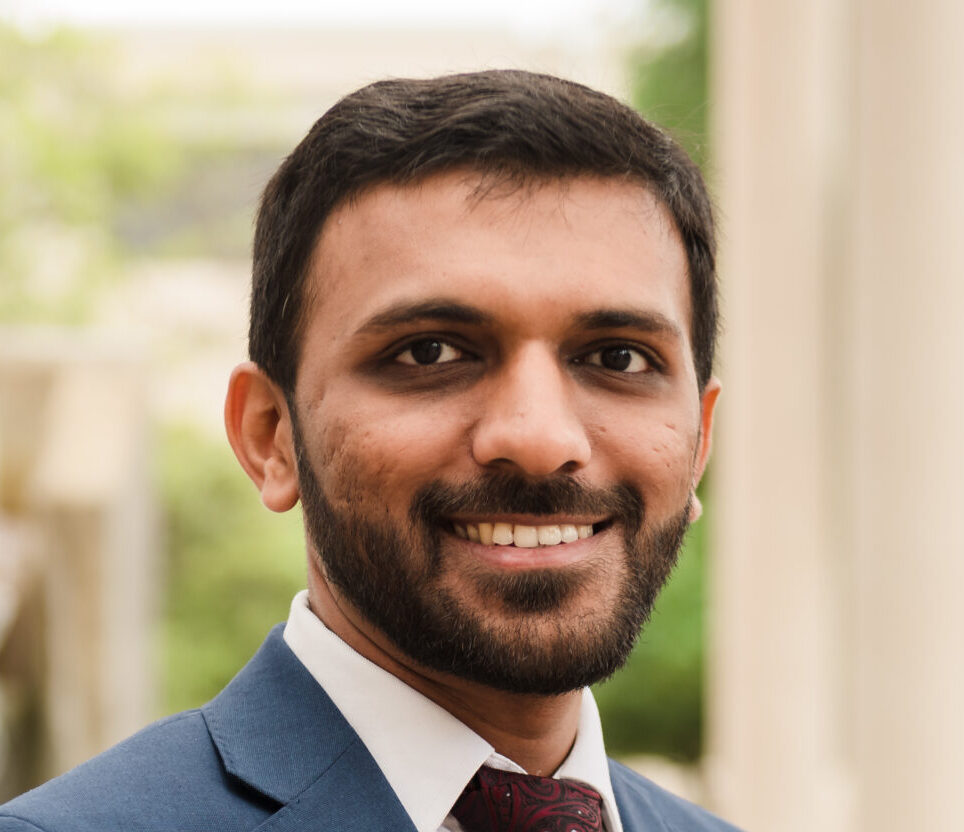
Institution: University of Texas at San Antonio
Biography:
Dr. Kevin Desai is an Assistant Professor of Instruction in the Computer Science department at the University of Texas at San Antonio. He received his PhD degree in Computer Science from The University of Texas at Dallas (UTD) in May 2019. He also received his MS in CS from UTD in May 2015, whereas his Bachelor of Technology in Computer Engineering from Nirma University (India) in June 2013. Dr. Desai’s research experience and interests are in the fields of Computer Vision and Immersive (Virtual / Augmented / Mixed) Realities with applications in the domains of healthcare, rehabilitation, virtual training, and serious gaming. He conducts interdisciplinary research which mainly revolves around the real-time capture and generation of 3D human models and their incorporation in collaborative 3D immersive environments. His research has been supported through various funding, specifically, the NSF CISE Research Initiation Initiative (CRII) award, one NSF small award, two NSF medium awards, and multiple other local / internal grants. Dr. Desai’s work has been published in peer-reviewed international conferences in the fields of computer vision (e.g., CVPRW, WACVW, ICIP, VISAPP), VR / AR / MR (e.g., VR, ISMAR, DIS), and Multimedia (e.g., MMSys, ISM, BigMM, ICME). He also serves as a program committee member and reviewer for top-tier international journals and conferences in IEEE, ACM, and Springer.
Schedule
Accommodations
If you plan to attend the NSF AI Spring School from a location outside San Antonio, we have compiled a list of hotels close to the venue to assist you in making your own arrangements. Note that this list is not exhaustive, and you are encouraged to explore other options based on your preferences and requirements.
Please be aware that participants are responsible for managing their own hotel bookings and cancellations.
The six listed hotels offer a discounted rate in partnership with UTSA.
Follow the instructions below to claim these rates while rooms are available.
More Hotels Nearby: 3 & 4-Star Options ▼
The Venue
Where to Park
You can park for free in any of the following lots:
Dolorosa Lot is the closest parking lot to San Pedro I bldg. You can park in any unmarked spot (Please do not park in spots labeled ‘Dolorosa Permit’).
D1, D2, & D3 Lots are located under I-10. UTSA permit holders may park in any unmarked spot.
More Parking Options
VIA Bus
Need transportation from main campus to downtown? You can board the VIA Bus!
UTSA Students can sign up for a free VIA bus pass here.
Board the 93 Bus for UTSA / Crossroads P&R / Downtown at the UTSA Campus Oval and get off at the Dolorosa Opposite Plaza De Armas stop (this stop is directly in front of the San Pedro I bldg).
Organizing Committee
Sponsors
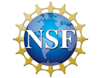
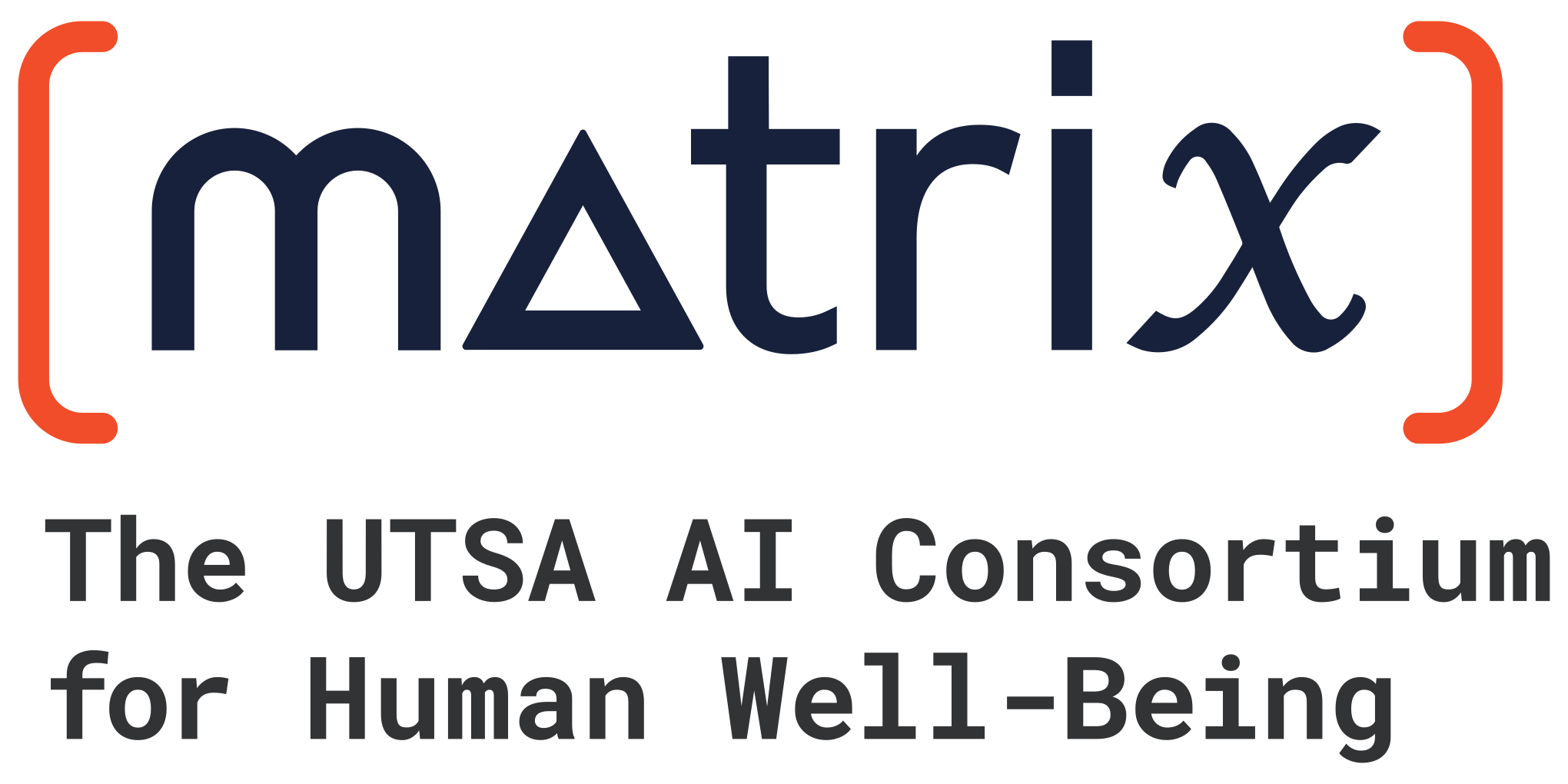
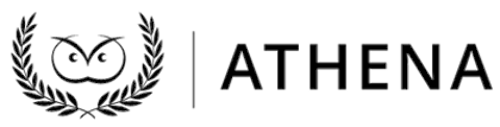
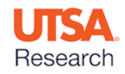
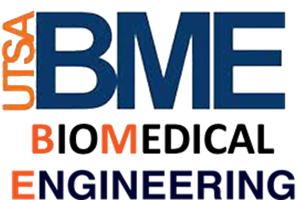
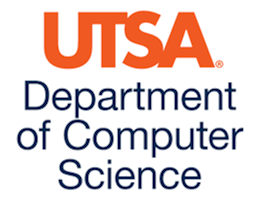